Short Courses
Deadline for short course registration: 10 July 12 August 2019 5:00pm JST Closed
Registration Fee for short course:20,000 JPY (Around US$ 180 ($1=109.78 as of March 25, 2019) ) / 1 short course
Please create a new account before short course registration.
With this account, you can log in to the system and enter "Short course registration" pages to start registration.
*The system is unavailable once the number of applicants reaches the capacity(24 People) of each course.

August 26(Mon) 9:30am-11:00am / Break / 11:15am-12:45pm
Missing Data Analysis in Clinical Trials Using SAS® <- Click here for details
Instructor (s): | G. Frank Liu, Merck, Sharp & Dohme, Corp; Fang Chen, SAS Institute Inc. |
Confirmatory Adaptive Designs with Multiple Objectives <- Click here for details
Instructor (s): | Frank Bretz, Novartis AG; Franz König, Medical University of Vienna; H.M. James Hung, Food and Drug Administration; Sue-Jane Wang, Food and Drug Administration; Martin Posch, Medical University of Vienna |
Accelerating Drug Discovery through Precision Medicine and Innovative Designs: Concepts, Rationale, and Case Studies <- Click here for details
Instructor (s): | Sandeep M Menon, Pfizer Inc., Boston University, Tufts University School of Medicine; Weidong Zhang, Pfizer Inc. |
August 26(Mon) 2:00pm-3:30pm / Break / 3:45pm-5:15pm
Novel Adaptive Clinical Trial Designs for Immunotherapy and Modern Drug Development <- Click here for details
Instructor (s): | Cong Chen, Merck & Co., Inc.; Guosheng Yin, University of Hong Kong; Ying Yuan, University of Texas MD Anderson Cancer Center |
Artificial Intelligence for Medicine and Health <- Click here for details
Instructor (s): | Mark Chang, Boston University |
Hot Topics in Clinical Trials: Multiple Outcomes and Benefit:risk <- Click here for details
Instructor (s): | Scott R. Evans, George Washington University; Toshimitsu Hamasaki, National Cerebral and Cardiovascular Center, Japan |
Missing Data Analysis in Clinical Trials Using SAS®
Instructor (s): | G. Frank Liu, Merck, Sharp & Dohme, Corp; Fang Chen, SAS Institute Inc. |
Missing data are inevitable and post many issues and challenge in analysis for clinical trials. Despite a great amount of research has been devoted to this topic, properly handling missing data in clinical trials remains complex. Conventionally, under the missing at random (MAR) assumption, we often use maximum likelihood or multiple imputation based methods for inferences. However, the MAR assumption is unverifiable. More critically, the estimand under MAR is hypothetical as indicated in the recent ICH E9 (R1) addendum and has been considered as overly-simplistic and unrealistic. Both regulatory agencies and industry sponsors have been seeking alternative approaches to handle missing data in clinical trials under missing not at random (MNAR) assumption.
This half-day tutorial is intended to cover issues of missing data in clinical trials including various methods and how to carry out the analyses using SAS software. The tutorial begins with an overview of missing data issues, and concepts and strategies as proposed by ICH E9 (R1) addendum. Then we will review traditional missing data handling methods such as maximum likelihood methods, multiple imputation, generalized estimation equation approaches, and Bayesian methods. The rest of the course is devoted to more recently-developed methods, such as sensitivity analysis to assess robustness, control-based imputation, control-based mean imputation, trimmed mean and tipping point analysis. Real clinical trial examples will be presented for illustration with implementation of the analysis using SAS/STAT software, including PROC MIXED, PROC MI, PROC MIANALYZE, PROC GEE, and PROC MCMC.
Outline
1. | Review missing data issues and ICH E-9 (R1) * Missing Data Issues in Clinical Trials * Review of ICH E9 (R1): Estimand and 5 Strategies |
2. | Traditional methods for missing data * Mixed-effects Model Repeated Measure (MMRM) * Constrained Longitudinal Data Analysis (cLDA) * GEE and wGEE * Multiple Imputation (MI) |
3. | Recently-developed methods under MNAR * Bayesian Methods * Control-based Imputation, Control-based Mean Imputation * Tipping Point Methods * Trimmed Mean Analysis |
About the Instructors
![]() |
Dr. G. Frank Liu is a distinguished scientist at Merck, Sharp & Dohme, Corp and a Fellow of the American Statistical Association (ASA). For more than 20 years at Merck, he has worked in a variety of therapeutic areas, including neuroscience, psychiatry, infectious disease, and vaccines. His research interests include methods for longitudinal trials, missing data, safety analysis, and noninferiority trials. He co-leads a Bayesian missing data analysis team in the DIA Bayesian Working Groups. He received a PhD in statistics from UCLA in 1994. |
![]() |
Dr. Fang Chen is a Director of Advanced Statistical Methods at SAS Institute Inc. Among his responsibilities are development of Bayesian analysis software and the MCMC procedure. He also oversees software development in various mixed models, nonlinear models, causal inferences, survival analysis, Bayesian hierarchical models, and discrete choice models. Before joining SAS, he received his PhD in statistics from Carnegie Mellon University in 2004. |
Confirmatory Adaptive Designs with Multiple Objectives
Instructor (s): | Frank Bretz, Novartis AG; Franz König, Medical University of Vienna; H.M. James Hung, Food and Drug Administration; Sue-Jane Wang, Food and Drug Administration; Martin Posch, Medical University of Vienna |
Adaptive (flexible) designs allow for mid-course design adaptations based on interim data without compromising the overall type I error rate. Examples of design adaptations are the adjustment of sample sizes or the number and timing of interim analyses. These design parameters may be adapted depending on interim estimates of the variance, the treatment effect and safety parameters. An important field of application of the adaptive design methodology are clinical trials with several treatment arms, where promising treatments can be selected at an interim analysis. Using adaptive multiple test procedures the type I error rate can be controlled even if the selection rule, the number of selected treatments or the final sample sizes are not prefixed. Adaptive multiple testing procedures can also be used in adaptive designs with the option of population enrichment. In such designs a sub population may be selected in an interim analysis and further recruitment of patients is restricted to the selected subgroup. In the past few years adaptations proposed in regulatory applications may involve a hybrid or a complex form of various design features, such as reasonably likely surrogate or predictive biomarker, external control. This short course will share with some regulatory experiences in such adaptive designs in cardiovascular, renal, CNS and imaging drug trials.
Adaptive Clinical Trial Designs
- Group sequential designs
- Adaptive combination tests
- Multiple testing in adaptive designs
- Case Studies
- Some regulatory experiences
About the Instructors
Franz König is Associate Professor at the Section of Medical Statistics at the Medical University of Vienna, Austria. He regularly serves as member of ethics committees and DSMBs. From 2008 till 2010 he was seconded to the European Medicines Agency as statistical expert. His main research interests are multiple testing and adaptive designs. See https://cemsiis.meduniwien.ac.at/user/koenig-franz/about-me/.
Martin Posch is professor of Medical Statistics at the Medical University of Vienna, Austria, and head of the Center for Medical Statistics, Informatics and Intelligent Systems. From 2011-2012 he worked as statistical expert at the European Medicines Agency. His research interests are group sequential trials, adaptive designs and multiple testing. See www.meduniwien.ac.at/user/martin.posch.
Frank Bretz joined Novartis in 2004, where he is currently Global Head of the Statistical Methodology and Consulting group. He has supported the methodological development in various areas of drug development, including dose-finding, multiple comparisons, and adaptive designs. He is an Adjunct Professor at the Hannover Medical School, Osaka University, the Shanghai University of Finance and Economics and the Medical University of Vienna.
H.M. James Hung is Director of Division of Biometrics I, Office of Biostatistics, OTS/CDER, US Food and Drug Administration.
Sue-Jane Wang is Associate Director, Office of Biostatistics and Acting Deputy Director, Division of Biometrics I, Office of Biostatistics, OTS/CDER US Food and Drug Administration.
Accelerating Drug Discovery through Precision Medicine and Innovative Designs: Concepts, Rationale, and Case Studies
Instructors: | Sandeep M Menon, Pfizer Inc., Boston University, Tufts University School of Medicine; Weidong Zhang, Pfizer Inc. |
Precision medicine has paved the way for a new era of delivering tailored treatment options to patients according to their biological profiles. Advancement of the biotechnologies such as next generation sequencing technology (NGS) and other omics technologies have enabled us to interrogate a patient's many molecular biomarkers, and associate them with disease and drug responses. In addition, incorporation of biomarker information in the innovative clinical trial design has presented drug developers unprecedented opportunities to bring a successful drug to patients in needs.
The first part of this course will focus on the concept of precision medicine, biomarker discovery and its application in clinical trials. Comprehensive review of omics data and major technologies will be presented. Statistical considerations and challenges such as data normalization, dimension reduction and biomarker threshold development and using biomarker for decision making in clinical development will be discussed in details.
The second part of this course will focus on the strategy of the study design that is important to critically determine biomarker performance, reliability and eventually regulatory acceptance. A general overview of the concept and statistical methodologies and designs related to precision medicine will be presented. Specifically, we will discuss various designs including adaptive designs available at our disposal and its merits and limitations.
About the Instructors
Dr. Sandeep Menon is currently the Vice President and the Head of Early Clinical Development Statistics at Pfizer Inc. and also holds Adjunct faculty positions at Boston University and Tufts University School of Medicine. He is an elected fellow of American Statistical Association.
Dr. Menon leads an organization of early development statisticians, clinicians and pharmacologists globally and is the executive member of the early clinical development leadership team and global clinical leadership team.
He is internationally known for his technical expertise especially in the area of adaptive designs, precision medicine, multi-regional trials, and small populations. He has participated in the core review of draft version of the regulatory guidance documents. He has co-authored and co-edited numerous books and contributed to influential papers in this area. He has taught short courses internationally and is a regular invited speaker in academia, FDA, Industry forums and Business Management institutes.
He is the co-author and co-editor of the books titled "Clinical and Statistical Considerations in Personalized Medicine","Modern Approaches to Clinical Trials Using SAS: Classical, Adaptive, and Bayesian Methods" and "Biosimilars – Clinical Development".
Sandeep received his medical degree from Bangalore (Karnataka) University, India, and later completed his Masters and Ph.D. in Biostatistics at Boston University and research assistantship at Harvard Clinical Research Institute. He has received several awards for academic excellence.
Dr. Weidong Zhang is a Senior Director in the Statistical Research and Innovation department at Pfizer. His responsibilities include statistical methodology development for precision medicine and providing scientific leadership and consultation in clinical biomarker strategy to senior Pfizer management and portfolios spanning from target discovery through proof-of-concept, and late phase studies across multiple therapeutic areas including Oncology, Immunology and inflammation, rare disease, and cardiovascular and metabolism. Weidong's research interest focuses on developing new statistical methods in biomarker discovery and precision medicine studies using high throughput omics data generated from cutting edge technologies including next-generation sequencing technology. He is passionate about teaching, and has taught short courses at many conferences including ASA Biopharmaceutical Section Regulatory-Industry Statistics Workshop, The Biopharmaceutical Applied Statistics Symposium (BASS) and the International Chinese Statistics Association (ICSA) Applied Statistics Symposium. He obtained his PhD degree in Statistical Genetics and MS degree in Statistics both from the University of Wisconsin-Madison.
Novel Adaptive Clinical Trial Designs for Immunotherapy and Modern Drug Development
Instructor (s): | Cong Chen, Merck & Co., Inc.; Guosheng Yin, University of Hong Kong; Ying Yuan, University of Texas MD Anderson Cancer Center |
Following the success of PD-1 (or PD-L1) inhibitors, a flood of next generation immunotherapies with different mechanisms of action are being developed. While the expectation is high for these new immunotherapies, it is unrealistic to expect all of them to have the same success as their predecessors, especially given the improved standard-of-care. Innovative adaptive clinical trial designs provide a cost-effective and flexible way to improve the success rate of drug development. In this short course, we will present novel Bayesian designs for phase I and II clinical trials (including both single-agent and drug-combination trials), statistical strategies on phase 1 efficacy screening, adaptive 2-in-1 design for seamless Phase 2/3, Phase 3 adaptive designs for population expansion. We will introduce the freely available software and illustrate the application of the designs using real-world examples. This short course is suitable for statisticians and clinicians from industry, regulatory agencies and academia. Students of this short course are expected to not only apply the new methods learned to their studies but also think out of box when facing unique situations.
About the Instructors
Dr. Ying Yuan is a Professor in the Department of Biostatistics at the MD Anderson Cancer Center. Dr. Yuan is an internationally renowned researcher in Bayesian adaptive clinical trial design, and was elected as ASA fellow in 2017. Dr. Yuan has proposed numerous innovative Bayesian adaptive designs for early phase trials, seamless trials, biomarker-guided trials, and basket and platform trials, which are increasingly used in practice. Dr. Yuan is the co-chief of the Adaptive Clinical Trial Design Section at MD Anderson Cancer Center, developing and overseeing novel adaptive clinical trials at the MD Anderson Cancer Center.
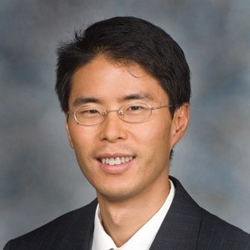
Professor Guosheng Yin is currently Patrick SC Poon Endowed Chair and Head of Department of Statistics and Actuarial Science at University of Hong Kong. He received Ph.D. in Biostatistics from University of North Carolina at Chapel Hill in 2003. He worked as Assistant Professor (2003-2008 tenure-track) and Associate Professor (2009 tenured) in the Department of Biostatistics at University of Texas M.D. Anderson Cancer Center. He joined HKU as Associate Professor since 2009, and was promoted to Professor in 2014. His main research areas include clinical trial methodology, adaptive design, deep learning, big data, data mining and machine learning, Bayesian methods, change-point and survival analysis. He has published over 140 peer-reviewed papers including top-tier statistical journals such as Journal of the American Statistical Association, Annals of Statistics, Biometrika, Biometrics, Annals of Applied Statistics etc. as well as top-tier medical journals including New England Journal of Medicine, Journal of Clinical Oncology, Journal of the National Cancer Institute etc. He single-authored one book "Clinical Trial Design: Bayesian and Frequentist Adaptive Methods" by the John Wiley Series in Probability and Statistics and coauthored another book "Statistical Design and Analysis in Clinical Trials" (in Chinese) by Higher Education Press. He co-taught a very popular short course on "Adaptive Methods in Modern Clinical Trials" in the largest statistical meeting JSM (Joint Statistical Meeting) in 2014 and 2015. He was among the world's top 1% of scientists ranked in one of the 22 subject fields of Thomson Reuters according to Essential Science Indicators in 2015. He was elected as a Fellow of the American Statistical Association in 2013, and elected as a Member of the International Statistical Institute in 2012. He received the James E. Grizzle Distinguished Alumni Award in the Department of Biostatistics, University of North Carolina at Chapel Hill. He was invited as a keynote speaker in multiple international workshops/meetings, and has given over 100 invited talks. He has served as Associate Editor for the journals including Statistical Analysis and Data Mining, Japanese Journal of Statistics and Data Science, Journal of American Statistical Association, Bayesian Analysis, and Contemporary Clinical Trials.
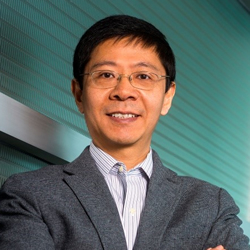
Dr. Cong Chen is Executive Director of Early Oncology Development Statistics at Merck & Co., Inc. He joined Merck in 1999 after graduating from Iowa State University with a Ph.D. in Statistics. He also holds a MS degree in Mathematics from Indiana University at Bloomington and a BS degree in Probability and Statistics from Beijing University, PR China.
As head of the group, he oversees the statistical support of oncology early clinical development at Merck. Prior to taking the role in March 2016, he led the statistical support for the development of pembrolizumab (KEYTRUDA), a paradigm changing anti-PD-1 immunotherapy, and played a pivotal role in accelerating its regulatory approvals.
He is a Fellow of American Statistical Association, an Associate Editor of Statistics in Biopharmaceutical Research, a member of Cancer Clinical Research Editorial Board and a co-leader of the DIA Small Population Work Stream. He has published over 70 papers and 9 book chapters on design and analysis of clinical trials, and was twice invited to give an oral presentation at the AACR Annual Meeting in recent years on oncology drug development.
Artificial Intelligence for Medicine and Health
Instructor (s): | Mark Chang, Boston University |
Artificial intelligence (AI) or machine learning (ML) has been used in drug development in biopharmaceutical companies for 20 years under name of bioinformatics, such as sequencing, annotating genomes, analysis of gene and protein expression and regulation, linking the biological and disease network to the symptoms and adverse events, identifying structure-activity relationships in discovery and designing new drugable molecules. AI has also been used for the prediction of cancer susceptibility (risk assessment), cancer recurrence/local control, and cancer survival. In analysis of clinical trial data, predicted individual patient outcomes for precision medicine, similarity-based machine learning (SBML) has recently been used in clinical trials for oncology and rare disease without the requirement of big data as most ML methods do. The course will cover: supervised, unsupervised, semi-supervised, and reinforcement learning methods, and swarm and evolutionary intelligences. It aims at conceptual clarity and mathematical simplicity. Provide R code for implementation and discuss over 20 real life examples in medicine and clinical trials, and a few other applications. Attendees will learn common AI methods in drug development and medicine, be able to use the AI methods with R for clinical trial and other data, and be able to interpret the results.
The course will be divided into three parts after introduction of a brief history AI in medicine and drug development:
(1) Supervised Learning (Regression & Classification)
Bayesian network
Nearest-Neighbors Method
Support Vector Machine
Tree-Based Methods
Artificial neural network & Deep Learning
R for AI
Application examples: disease diagnosis, prognosis, and survival prediction
(3) Similarity Based Method:
Kernel Method
The Similarity Principle
Similarity-based machine learning
Sequential and Recursive SBMLs
R programs
Clinical Trial and other examples
(4) Other AI methods and Future Perspectives
Unsupervised Learning
Semi-supervised learning
Reinforcement Learning,
Swarm Intelligence
Evolutionary Intelligence (GP)
Future perspectives
About the Instructors
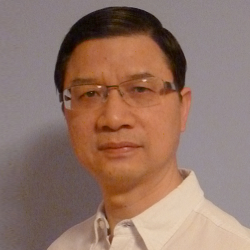
Dr. Mark Chang is founder of AGInception, a research organization for artificial general intelligence. He was Sr. Vice President, Strategic Statistical Consulting at Veristat and Vice President of Biometrics at AMAG Pharmaceuticals. Chang is a fellow of the American Statistical Association and an adjunct professor of Biostatistics at Boston University. He is a co-founder of the International Society for Biopharmaceutical Statistics, co-chair of the Biotechnology Industry Organization (BIO) Adaptive Design Working Group, and a member of the Multiregional Clinical Trial (MRCT) Expert Group. Chang has served associate editor for Journal of Pharmaceutical Statistics. Dr. Chang has published 10 books, including Adaptive Design Theory and Implementation Using SAS and R, Paradoxes in Scientific Inferences, Modern Issues and Methods in Biostatistics, Monte Carlo Simulation for the Pharmaceutical Industry, Principles of Scientific Methods, and Innovative Strategies, Statistical Solutions and Simulations for Modern Clinical Trials.
Hot Topics in Clinical Trials: Multiple Outcomes and Benefit:risk
Instructor (s): | Scott R. Evans, George Washington University; Toshimitsu Hamasaki, National Cerebral and Cardiovascular Center, Japan |
We discuss two hot topics in clinical trials. In Part I we discuss the design and analysis of clinical trials with multiple outcomes. In Part II, we discuss benefit:risk evaluation in clinical trials by using outcomes to analyze patient rather than patients to analyze outcomes.
PART I: The effects of interventions are multidimensional. Use of more than one outcome offers an attractive design feature in clinical trials as they capture more complete characterization of the benefit and risk of an intervention and provide more informative intervention comparisons. The tutorial will focus on design and analysis of clinical trials with such multiple outcomes. The first part of the tutorial will focus on methods for clinical trial designs evaluating efficacy of two interventions with multiple primary endpoints, especially co-primary endpoints. "Co-primary" means that a trial is designed to evaluate if the test intervention is superior (or noninferior) to the control on all primary endpoints. We describe methods for power and sample size calculations in clinical trials with multiple endpoints including recently developed approaches. We include real clinical trial examples to illustrate the concepts and to help participants apply the methods in practice, and illustrate how to implement the methods using standard statistical software including R and SAS.
PART 2: In the future, clinical trials will have an increased emphasis on pragmatism, providing a practical description of the effects of new treatments in realistic clinical settings. Accomplishing pragmatism requires better summaries of the totality of the evidence that allow for informed benefit:risk decision-making and in a way that clinical trials consumers—patients, physicians, insurers—find transparent. The current approach to the analysis of clinical trials is to analyze efficacy and safety separately and then combine these analyses into a benefit:risk assessment. Many assume that this will effectively describe the impact on patients. But this approach is suboptimal for evaluating the totality of effects on patients. In part II of the tutorial, we will describe a broad vision for the future of clinical trials consistent with increased pragmatism. Greater focus on using outcomes to analyze patients rather than patients to analyze outcomes particularly in late-phase/stage clinical trials is an important part of this vision. We discuss the desirability of outcome ranking (DOOR) and the partial credit strategy for design and analysis of clinical trials based on benefit:risk assessment. These strategies involve utilizing composite benefit:risk endpoints with a goal of understanding how to analyze one patient before trying to figure out how to analyze many. With a desire to measure and weigh outcomes that are most important from the patient's perspective, we discuss using patients as a resource to inform analyses.
About the Instructors
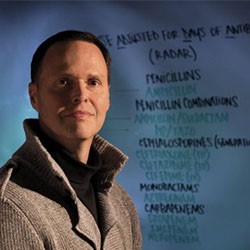
Dr. Scott Evans is a Professor of Epidemiology and Biostatistics and the Director of the George Washington Biostatistics Center. Professor Evans interests include the design, monitoring, analyses, and reporting of and education in clinical trials and diagnostic studies. He is the author of more than 100 peer-reviewed publications and three books on clinical trials including Fundamentals for New Clinical Trialists. He is the Director of the Statistical and Data Management Center (SDMC) for the Antibacterial Resistance Leadership Group (ARLG), a collaborative clinical research network that prioritizes, designs, and executes clinical research to reduce the public health threat of antibacterial resistance.
Professor Evans is a member of the Board of Directors for the American Statistical Association (ASA) and formerly for the Society for Clinical Trials (SCT) and the Mu Sigma Rho (the National Honorary Society for Statistics). He is a member of an FDA Advisory Committee, the Steering Committee of the Clinical Trials Transformation Initiative (CTTI), the Executive Committee for the Analgesic, Anesthetic, and Addiction Clinical Trial Translations, Innovations, and Networks (ACTTION), and serves as the Chair of the Trial of the Year Committee of the SCT.
Professor Evans is the Editor-in-Chief of CHANCE and Statistical Communications in Infectious Diseases (SCID), and the Co-Editor of a Special Section of Clinical Infectious Diseases (CID) entitled Innovations in Design, Education, and Analysis (IDEA).
Dr. Evans is a recipient of the Mosteller Statistician of the Year Award, the Robert Zackin Distinguished Collaborative Statistician Award for contributions to the AIDS Clinical Trials Group (ACTG), an elected member of the International Statistical Institute (ISI), and is a Fellow of the American Statistical Association (ASA), Society for Clinical Trials (SCT), and the Infectious Disease Society of America (IDSA).
Dr. Toshimitsu Hamasaki is the Director of Data Science at National Cerebral and Cardiovascular Center (NCVC), Osaka, Japan. He has been involved in biopharmaceutical statistics for over 25 years, and prior to joining NCVC, worked at Shiogoni, Pfizer Japan and Osaka University. He has been actively involved in biostatistical research, and is the author of more than 180 peer-reviewed publications and three textbooks on clinical trials including Group-Sequential Clinical Trials with Multiple Co-Objectives. Dr. Hamasaki was the member of ICH E5 Guideline Implementation Working Group as a representative of Japan Pharmaceutical Manufacturers Association to develop the Q & A document on the guideline. He currently serves as an Associate Editor for Statistics in Biopharmaceutical Research and Journal of Biopharmaceutical Statistics, and Editor for CHANCE. He is an elected member of International Statistical Institute and a Fellow of the American Statistical Association He is a recipient of the Japanese Society of Computational Statistics Distinguished Article Award and Behaviormetric Society of Japan Hida-Mizuno Prize, and the Poster Competition First Place Winner at the ASA Biopharmaceutical Section Regulatory-Industry Statistics Workshop.